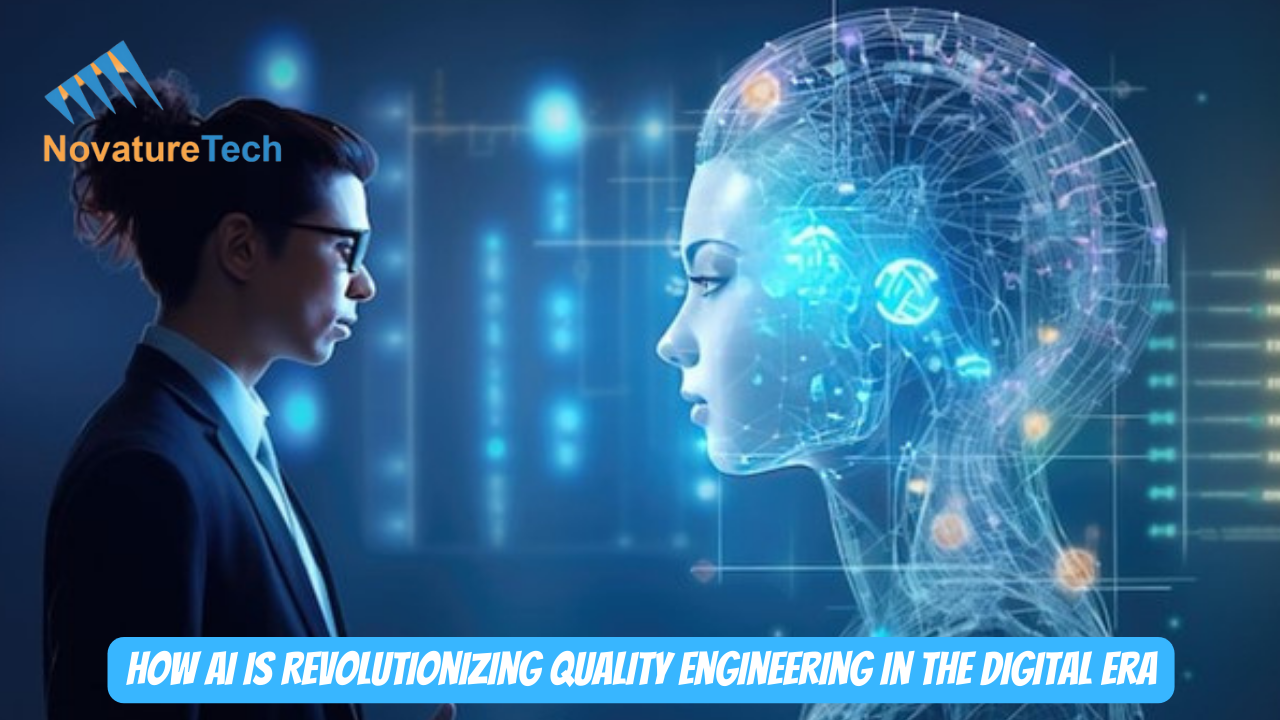



How AI is Revolutionizing Quality Engineering in the Digital Era
Table of Contents:
Introduction
The Benefits of AI in Quality Engineering
The Current State of AI in Quality Engineering
The Future of AI in Quality Engineering
The Role of Developers and Testers in an AI World
Conclusion
Introduction
In today’s rapidly evolving digital landscape, businesses must continually innovate and adapt to meet the ever-changing demands of customers. Quality Engineering (QE) plays a crucial role in ensuring that software applications meet the highest standards of performance, security, and user experience. With the advent of Artificial Intelligence (AI), QE is undergoing a transformative shift, enabling organizations to achieve digital transformation and deliver exceptional software products. This article explores the rise of AI in Quality Engineering and its impact on software testing.
AI brings numerous benefits to the field of Quality Engineering, revolutionizing the way software testing is conducted. Let’s explore some of the key advantages:
Test automation has long been a fundamental aspect of Quality Engineering, enabling the execution of repetitive test scenarios quickly and consistently. With AI, test automation reaches new heights. AI-driven testing tools can intelligently identify test cases that require automation, automatically generate scripts, and adapt to changes in the application’s user interface. This dynamic and adaptive approach accelerates the testing process, leading to increased efficiency and faster development cycles.
In large-scale software projects, the number of test cases can be overwhelming. AI can analyze various factors, such as business impact, risk assessment, and historical defect patterns, to intelligently prioritize test cases. This ensures that limited resources are allocated optimally, maximizing test coverage and minimizing the risk of critical defects slipping through the cracks.
By analyzing historical defect data, AI can identify patterns and predict potential defect-prone areas in an application. This proactive approach allows Quality Engineering teams to address these areas early in the development process, leading to improved software quality and reduced post-release defects. AI-powered defect prediction empowers organizations to deliver more reliable software products.
Writing test scripts traditionally requires technical expertise and can be time-consuming. AI-driven Natural Language Processing (NLP) technologies revolutionize test scripting by enabling testers to write test cases in natural language, which are then automatically converted into executable test scripts. This simplifies the testing process, encourages collaboration between technical and non-technical stakeholders, and enhances overall efficiency.
AI-powered chatbots can be leveraged for cognitive testing, simulating real-world scenarios and interacting with applications as human users would. This approach uncovers potential issues, ensures that applications meet user expectations, and enhances the overall quality of the software. Cognitive testing with AI chatbots provides valuable insights into the user experience and identifies areas for improvement.
Performance testing is crucial for identifying bottlenecks and ensuring that applications can handle varying workloads. AI can simulate complex user behavior and generate virtual users, allowing Quality Engineering teams to conduct comprehensive and accurate performance tests. AI-driven performance testing enables organizations to optimize the scalability, responsiveness, and overall performance of their software applications.
While AI holds immense potential in Quality Engineering, its adoption is still in its early stages. Organizations are beginning to explore and integrate AI-driven tools and services into their software testing processes, but there is still room for growth and innovation. However, several real-world examples demonstrate the practical application of AI in Quality Engineering:
AI-based tools, such as Sapienz developed by Facebook, can automatically generate test scenarios based on the application under test. These tools significantly reduce the time and effort required to build and maintain test cases, enabling Quality Engineering teams to focus on more critical tasks and improve overall efficiency.
Visual testing, which ensures that an application’s user interface looks as intended across different devices, browsers, and screen resolutions, can be challenging and time-consuming. AI algorithms, employed by platforms like Applitools, improve the analysis of an application’s content, saving time by detecting significant differences while ignoring minor changes. This enhances the accuracy and reliability of visual regression testing.
Setting up test automation projects often requires significant upfront time and effort. AI-powered tools, such as GitHub Copilot, automate the process by generating boilerplate code, allowing Quality Engineering teams to focus on more critical aspects of testing and accelerating the development process.
In the fast-paced world of software development, codebase changes occur frequently. AI-powered testing tools, like Telerik Test Studio, leverage AI processes to help testers adjust existing test scenarios quickly and accurately. These tools detect test failure reasons and provide solutions, reducing the time spent fixing broken tests and improving overall efficiency.
While the current state of AI in Quality Engineering is still evolving, these real-world examples demonstrate its practical application and potential to transform software testing processes.
As AI continues to advance, its role in Quality Engineering is expected to expand further. The future holds several exciting trends and possibilities:
Integrating AI into existing Quality Engineering processes and workflows will be a primary focus in the future. Organizations, like GitLab, are already exploring the use of AI and machine learning to enhance their DevOps and security platforms. The seamless integration of AI into existing testing practices will drive widespread adoption and enable organizations to leverage AI’s full potential.
Accessibility is becoming increasingly important in software development, ensuring that applications are accessible to all users, regardless of their physical abilities. AI can play a significant role in assisting Quality Engineering teams in making applications fully accessible. Companies like Deque Systems are already using AI to develop tools that help make software accessible to all, reinforcing the importance of AI in driving inclusivity in software development.
Ensuring optimal performance and reliability of software systems after deployment is crucial. AI can automate monitoring and observability processes, allowing engineers to identify and adjust metrics or rules in real-time. AI-powered systems can help site reliability engineers keep monitored systems running at peak condition by providing insights and proactive solutions.
These trends represent just a fraction of the potential AI holds in Quality Engineering. As AI technologies mature and organizations embrace their capabilities, the impact on software testing will continue to grow, driving innovation and delivering higher-quality software products.
With the rise of AI in Quality Engineering, concerns about job security among developers and testers have surfaced. However, AI is not intended to replace human testers or developers. While AI-powered tools and services enhance certain aspects of testing, human expertise, experience, and reasoning remain essential. AI is not infallible and can still make mistakes. Organizations that rely solely on AI without human oversight are likely to encounter problems.
Developers and testers excel in areas such as experience, reasoning, and understanding the context of complex systems. While AI can improve efficiency and accuracy, it is unlikely to reach the level of human expertise anytime soon. Developers and testers should embrace AI as a tool to enhance their work rather than fear it as a replacement.
As AI continues to evolve, developers and testers should focus on upskilling and expanding their knowledge to work alongside AI-powered tools and services. By leveraging the strengths of both AI and human expertise, organizations can achieve optimal results and deliver high-quality software in the digital era.
AI is revolutionizing Quality Engineering in the digital era, transforming the way software testing is conducted. From enhanced test automation and intelligent test case prioritization to AI-enabled defect prediction and cognitive testing with AI chatbots, the benefits of AI in Quality Engineering are extensive. While the current state of AI adoption in Quality Engineering is still evolving, real-world examples demonstrate its practical application and potential.
As AI technologies mature and organizations integrate AI into their testing processes, we can expect to see even greater advancements in Quality Engineering. The future holds exciting possibilities, including better integration with existing processes, improved accessibility, and hands-off monitoring and observability. Developers and testers must embrace AI as an ally rather than a threat, leveraging its capabilities to enhance their work and deliver exceptional software products.
In this rapidly evolving digital landscape, organizations must prioritize AI in Quality Engineering to stay ahead of the competition and achieve digital transformation. By harnessing the power of AI, businesses can drive innovation, improve efficiency, and deliver high-quality software that meets the evolving needs of customers. Embrace AI in Quality Engineering and unlock the full potential of software testing in the digital era.
In conclusion, Novature Tech is at the forefront of AI-based Quality Engineering, leveraging advanced tools like Sapienz, Applitools, GitHub Copilot, and Telerik Test Studio. Specializing in AI-driven test automation, intelligent prioritization, and defect prediction, we propel clients towards accelerated development cycles and enhanced software quality. Positioned for the future, we envision seamless AI integration, improved accessibility, and hands-off monitoring. Novature Tech invites businesses to embrace AI in Quality Engineering, ensuring digital transformation, competitiveness, and unparalleled software quality. With us, clients unlock AI’s full potential, setting new standards for innovation in the dynamic technological landscape. Choose Novature Tech for a future-driven approach to software testing excellence.
Author: admin | Posted On: 16th January 2024 | Category: Article
© 2024 Novature Tech Pvt Ltd. All Rights Reserved.